SIS Next-Level-Welfare
Next-Level-Welfare Behavioural monitoring of aquatic animals
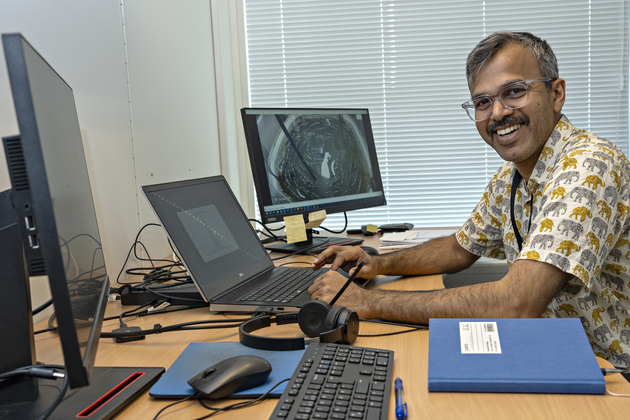
The project aims to advance aquaculture through digital innovation by developing a robust ML and IT infrastructure, diversifying digital tool applications, refining behavioural analysis models, conducting a SWOT analysis, and disseminating knowledge through publications and guidelines.
Start
01. Jan 2025
End
24. Dec 2027
Funded by
Nofima
Project Manager(s):
Background
Precision Fish Farming is transforming aquaculture by addressing the underutilization of behavioral monitoring as a welfare indicator, leveraging AI and ML to enhance animal welfare and health documentation, optimize sustainable production, and foster new research collaborations, thereby solving the problem of inadequate and manual auditing methods that limit the utility and applicability of behavioral data.
Objectives
Boosting Nofima’s position and capabilities regarding Behavioural assessments in aquaculture and fisheries using AI:
- Develop ML and IT platforms: Create portable camera solutions and manage video datasets for systematic behavioral analysis.
- Diversify digital tools: Study different life stages, rearing systems, and their relevance to fish health and climate change.
- Enhance the digital toolbox: Improve behavior analysis models, use data from sensors like sonar, and integrate into a comprehensive toolbox.
How we work
Building a robust dataset is the first step towards leveraging AI in aquaculture research at Nofima. This dataset will serve as the foundation for developing generalized machine learning models to quantify behaviours.
A well-maintained dataset can be applied in various contexts, such as understanding climate impacts, assessing health threats, and optimizing rearing systems.
We tag along the experimental trials in 2025 and 2026 to videotape the behavioural aspects. We will develop and refine our digital toolbox by enhancing existing deep learning and classical models for behaviour analysis, exploiting data from other sensors (e.g., sonar), and integrating data into a comprehensive toolbox for fish behavioural analysis.
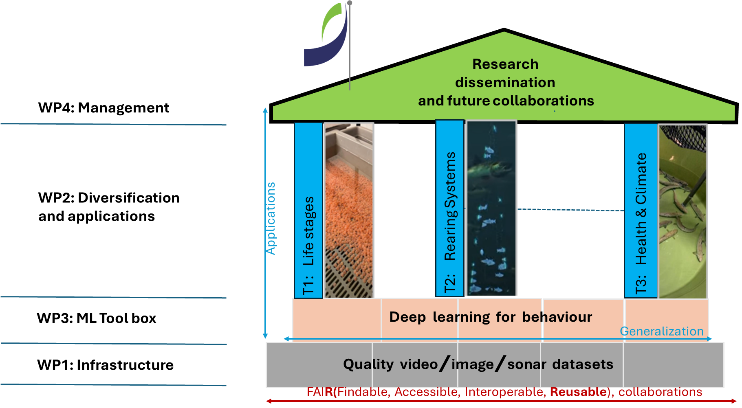
The findings from these studies can be disseminated through publications and presentations, fostering collaborations with other researchers and organizations. Moreover, a well-structured and accessible dataset promotes the FAIR (Findable, Accessible, Interoperable, Reusable) principles, facilitating collaboration and knowledge sharing.
Strategic priority areas
Nofima invests its own resources in order to increase competence in useful, relevant and innovative areas and strengthen our position among the leading applied research institutes.
Active projects
Some previous projects
Research areas
Farmed fish
Topics
Fish welfare
Similar projects